Previously, we described the essentials of R programming and provided quick start guides for importing data into R. The next crucial step is to set your data into a consistent data structure for easier analyses. Here, you’ll learn modern conventions for preparing and reshapingdata in order to facilitate analyses in R.
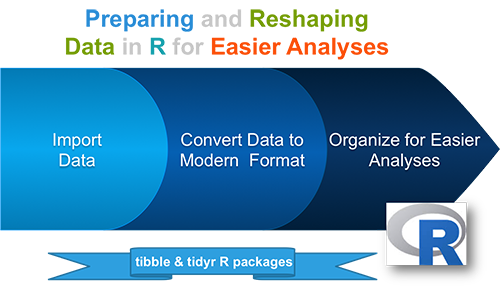
- Installing and loading tibble package: type install.packages(“tibble”) for installing and library(“tibble”) for loading.
- Create a new tibble: data_frame(x = rnorm(100), y = rnorm(100)).
- Convert your data as a tibble: as_data_frame(iris)
- Advantages of tibbles compared to data frames: nice printing methods for large data sets, specification of column types.
Read more: Tibble Data Format in R: Best and Modern Way to Work with your Data
- What is a tidy data set?: a data structure convention where each column is a variable and each row an observation
- Reshaping data using tidyr package
- Installing and loading tidyr: type install.packages(“tidyr”) for installing and library(“tidyr”) for loading.
- Example data sets: USArrests
- gather(): collapse columns into rows
- spread(): spread two columns into multiple columns
- unite(): Unite multiple columns into one
- separate(): separate one column into multiple
- %>%: Chaining multiple operations
Read more: Tidyr: crucial Step Reshaping Data with R for Easier Analyses